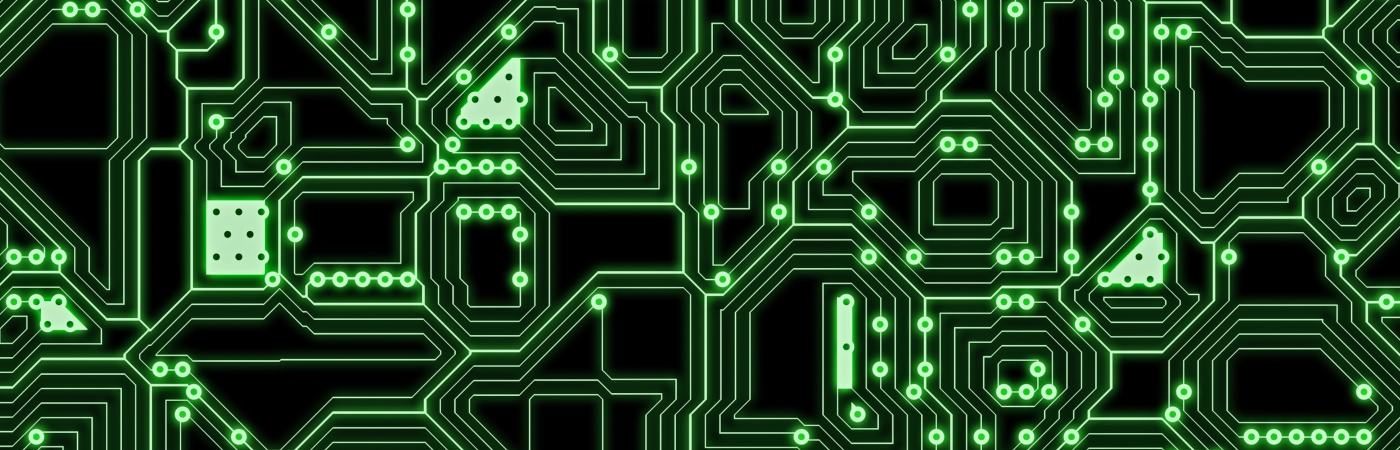
University of Manchester scientists have developed a form of machine learning which could dramatically reduce the numbers of animals needed by medical researchers.
Data scientists Dr Paul Tar and Dr Neil Thacker say the technique could mean researchers need up to 16 times fewer animals to accurately assess potential treatments using medical imaging.
The technique has only been demonstrated using mice so far, but the method could be applied to measure the effects of interventions in different animals.
Called Linear Poisson Modelling, the technique is a type of machine learning for making efficient use of complex data.
“The standard format of statistical modelling, the T Test, was developed long before computers to crunch small amounts of data, said Dr Tar.
“But the problem with modern data sets is that they are complicated. Happily, computing allows us to go back to first principles which is where Linear Poisson Modelling comes in.
“As long as the data can be presented in the form of histograms in an appropriate format, the method can be used in a wide variety of applications.
“The treatment effect measurements have only be tested in cancers so far, but potentially could be applied in other diseases too. In fact, our aim is to turn our experimental software that can be used more widely.”
The development also means that up to five times less biological material is required for making measurements using mass spectroscopy techniques.
It opens up opportunities for new experimental designs that can increase scientific outputs while using as few animals as possible.
Dr Paul Tar / Data scientist
Statistical techniques
Machine learning uses statistical techniques to give computer systems the ability to "learn" without being explicitly programmed. The improved modelling of data means that fewer samples are needed to provide highly accurate results.
In recognition of the contribution of the Manchester team of researchers from the fields of machine learning, medicine and the life sciences, they were named in October 2018 as winners the TurnKey Innovation Award.
Originally developed by Dr Tar and Dr Thacker 9 years ago to map Mars, the machine learning algorithm has proven to be highly useful in other areas, from biochemistry to cancer studies.
Dr Tar said: “This technique is all about making the most of ‘small data’, which is common in medical studies where it is difficult to obtain large numbers of samples.
“It’s also about utilising advances in imaging techniques, including mass spectra imaging, which can extract more information per animal.
“It opens up opportunities for new experimental designs that can increase scientific outputs while using as few animals as possible.”
Experimental designs
He added: “The most obvious benefit of the new approach is in the reduction of animal numbers in research, but opportunities exist to refine and in some circumstances to aid the replacement of animals through developing a better understanding of the behaviour of complex data.
“A combination of tumour imaging followed by an analysis of tumour sections via mass spectrometry, for example, could provide valuable insights for informing future non-animal models and corroborate their predictions.
“The method opens up possibilities for new experimental designs that could allow these benefits to be fully realized.”
Dr Thacker said: “It’s incredible that statistically significant results can be achieved with only a single treated tumour in a single mouse, as a sample size of one contains very little information.
“But there is far more than just one piece of information within each object. If this wasn't true, for example, then it would be impossible to find your own car in a crowded car park.
“Unlike other popular machine learning technologies, such as Deep Learning, our approach directly incorporates knowledge of how certain aspects of data behave, so that there's less to learn.
“These gains open opportunities for new experimental designs that can increase scientific outputs while using as few animals as possible.”