Overview
- Degree awarded
- MSc
- Duration
- 12 months full time
- Entry requirements
-
The normal entry requirements are a good upper second or first class honours degree from a UK university, or the equivalent from an overseas university, in Mathematics or in a subject with some significant mathematical content. Students should also have some knowledge of Probability and Statistics in their degree, at least up to what might be expected by the end of the second year of an undergraduate Mathematics degree.
Expected Background
You are expected to have a first degree with a substantial amount of mathematics including Probability and Statistics. As a minimum you should have done Calculus or Mathematical Analysis, Linear Algebra, two courses in Probability and two courses in Statistics. A Mathematical Statistics course may count as one Probability and one Statistics course depending on the syllabus. If your course is called Advanced Mathematics, then we need to know how much calculus/linear algebra it contains. You can have a look at what Manchester students do in the first two years, or refer to the following list for a quick summary.
- Calculus or Mathematical Analysis (functions of a single and several variables, continuity, derivatives, integrals, Mean Value Theorem, Taylor series expansion, minimisation and maximisation, Lagrange multipliers)
- Linear Algebra (linear independence, determinant, inverse, eigenvalues and eigenvectors)
- Probability I (probabilities and conditional probabilities, Bayes Theorem, moments)
- Probability II (multivariate and conditional distributions, generating functions, Law of Large Numbers and Central Limit Theorem)
- Statistics I (descriptive statistics, normal, t, chi-square and F distributions, significance tests)
- Statistics II (Maximum likelihood estimation, Likelihood ratio tests, simple regression and analysis of variance).
You are expected to have done well in the above courses and your university should have a high national standing.
- How to apply
-
As there is high demand for this course we operate a staged admissions process with selection deadlines throughout the year.
Due to the competition for places, we give preference to students with grades above our minimum entry requirements. If we make you an offer, you will have 3 weeks in which to accept. Any offers not accepted within 3 weeks will be withdrawn so that an offer can be made to another candidate.
Please find more information in our Application and Selection section .
- Sustainable Development Goals
Find out how this course aligns to the UN Sustainable Development Goals, including learning which relates to:
- Goal 4: Quality education
Course options
Full-time | Part-time | Full-time distance learning | Part-time distance learning | |
---|---|---|---|---|
MSc | Y | Y | N | N |
Course description
The Probability and Statistics group in the Department of Mathematics have a long-standing reputation of offering one-year, high-quality taught courses in areas of Statistics leading to the degree of MSc. These courses have aimed to offer a thorough professional training which prepare students to embark on statistical careers in a variety of areas.
There is a shortage of statisticians trained to postgraduate level in the UK, meaning that you will be a highly desired candidate upon completion of this MSc. They have also provided a very good foundation for further study at PhD level.
Open days
Fees
For entry in the academic year beginning September 2025, the tuition fees are as follows:
-
MSc (full-time)
UK students (per annum): £15,500
International, including EU, students (per annum): £34,500 -
MSc (part-time)
UK students (per annum): £8,000
International, including EU, students (per annum): £16,500
Further information for EU students can be found on our dedicated EU page.
The fees quoted above will be fully inclusive for the course tuition, administration and computational costs during your studies.
All fees for entry will be subject to yearly review and incremental rises per annum are also likely over the duration of courses lasting more than a year for UK/EU students (fees are typically fixed for International students, for the course duration at the year of entry). For general fees information please visit: postgraduate fees . Always contact the department if you are unsure which fee applies to your qualification award and method of attendance.
Self-funded international applicants for this course will be required to pay a deposit of £1000 towards their tuition fees before a confirmation of acceptance for studies (CAS) is issued. This deposit will only be refunded if immigration permission is refused. We will notify you about how and when to make this payment.
Policy on additional costs
All students should normally be able to complete their programme of study without incurring additional study costs over and above the tuition fee for that programme. Any unavoidable additional compulsory costs totalling more than 1% of the annual home undergraduate fee per annum, regardless of whether the programme in question is undergraduate or postgraduate taught, will be made clear to you at the point of application. Further information can be found in the University's Policy on additional costs incurred by students on undergraduate and postgraduate taught programmes (PDF document, 91KB).
Scholarships/sponsorships
Across our institution, we offer a number of postgraduate taught scholarships and awards to outstanding UK and international students each year. We are one of the Martingale Foundation's newest university partners. The Martingale Foundation helps provide access to postgraduate mathematics study for UK students facing financial barriers by offering fully-funded MSc and PhD programmes. Information about eligibility is available via the Martingale Foundation website and applications will open on 13 August 2025. The deadine to apply is Sunday 19 October 2025. For more information, see the Department ofMathematics Fees and funding page orvisit the University of Manchester funding for master's courses website for more information.
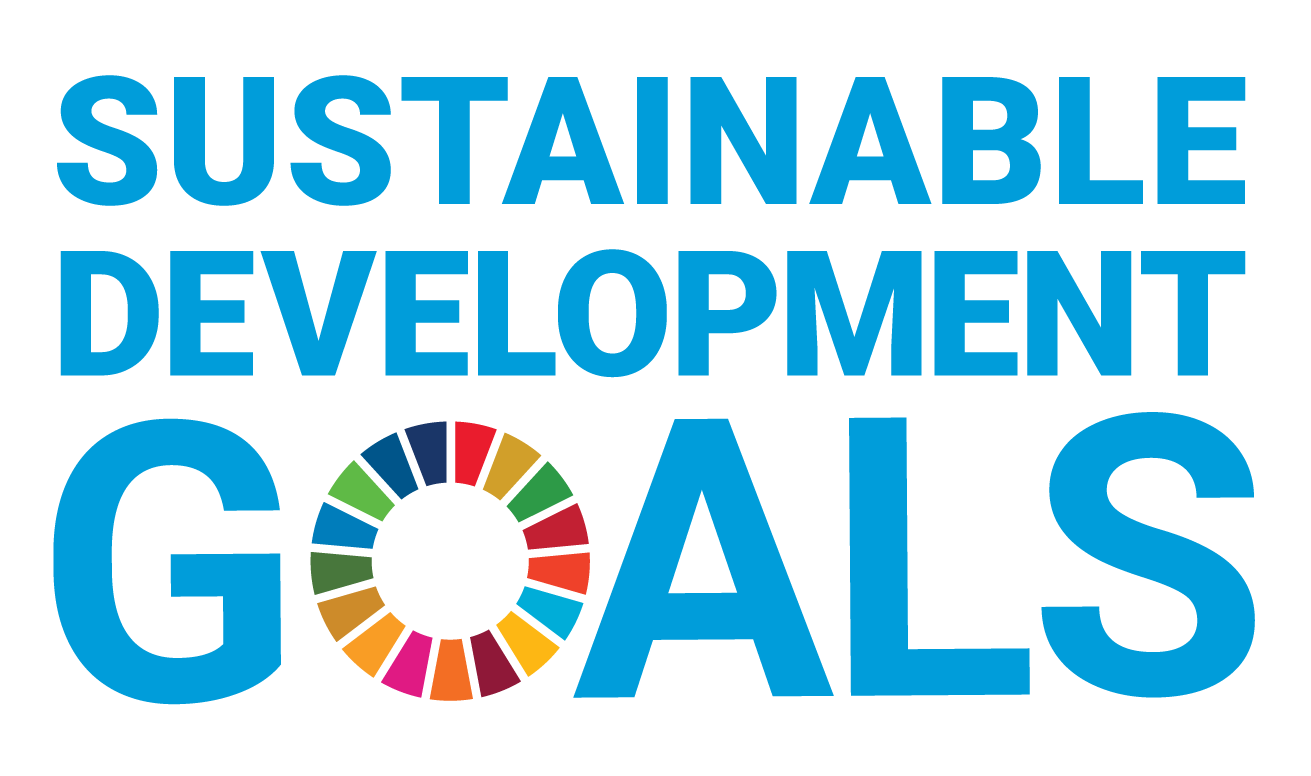
UN Sustainable Development Goals
The 17 United Nations Sustainable Development Goals (SDGs) are the world's call to action on the most pressing challenges facing humanity. At The University of Manchester, we address the SDGs through our research and particularly in partnership with our students.
Led by our innovative research, our teaching ensures that all our graduates are empowered, inspired and equipped to address the key socio-political and environmental challenges facing the world.
To illustrate how our teaching will empower you as a change maker, we've highlighted the key SDGs that our courses address.
Goal 4: Quality education
Ensure inclusive and equitable quality education and promote lifelong learning opportunities for all
Contact details
- Department
- Department of Mathematics
- Contact name
- Postgraduate Admissions Team
- Telephone
- +44 (0)161 529 3043
- pgt-maths@manchester.ac.uk
- Website
- https://www.manchester.ac.uk/maths
- School/Faculty
-
Our internationally-renowned expertise across the School of Natural Sciences informs research led teaching with strong collaboration across disciplines, unlocking new and exciting fields and translating science into reality. Our multidisciplinary learning and research activities advance the boundaries of science for the wider benefit of society, inspiring students to promote positive change through educating future leaders in the true fundamentals of science. Find out more about Science and Engineering at Manchester .
Courses in related subject areas
Use the links below to view lists of courses in related subject areas.
Entry requirements
Academic entry qualification overview
Expected Background
You are expected to have a first degree with a substantial amount of mathematics including Probability and Statistics. As a minimum you should have done Calculus or Mathematical Analysis, Linear Algebra, two courses in Probability and two courses in Statistics. A Mathematical Statistics course may count as one Probability and one Statistics course depending on the syllabus. If your course is called Advanced Mathematics, then we need to know how much calculus/linear algebra it contains. You can have a look at what Manchester students do in the first two years, or refer to the following list for a quick summary.
- Calculus or Mathematical Analysis (functions of a single and several variables, continuity, derivatives, integrals, Mean Value Theorem, Taylor series expansion, minimisation and maximisation, Lagrange multipliers)
- Linear Algebra (linear independence, determinant, inverse, eigenvalues and eigenvectors)
- Probability I (probabilities and conditional probabilities, Bayes Theorem, moments)
- Probability II (multivariate and conditional distributions, generating functions, Law of Large Numbers and Central Limit Theorem)
- Statistics I (descriptive statistics, normal, t, chi-square and F distributions, significance tests)
- Statistics II (Maximum likelihood estimation, Likelihood ratio tests, simple regression and analysis of variance).
You are expected to have done well in the above courses and your university should have a high national standing.
English language
All applicants will need to demonstrate competency in English language. Applicants who do not already possess an acceptable English Language qualification will need to take a recognised test and attain the required English Language score:
- IELTS: at least 6.5 overall with 6.5 in writing and no other sub-test less than 6.0.
- TOEFL iBT: at least 90 overall with 22 or above in writing and no other sub-test less than 20. We do not accept 'MyBestScore'.
- Pearson PTE: at least 70 overall with 70 in writing and no other sub-test below 65.
Further information on language requirements can be found on our website .
Pre-sessional English
We will consider applicants who do not meet these scores but you may be required to complete a pre-sessional English language course at the University of Manchester prior to the start of the course.
To be considered for a pre-sessional English language course for this programme we require the following minimum IELTS (Academic) scores:
- 6 Week Pre-Sessional Course: Minimum 6.0 overall with 6.0 in three sub-tests including writing and at least 5.5 in the remaining sub-test.
If you have not yet completed your current academic study and are interested in studying a pre-sessional course, you must hold an IELTS for UKVI (Academic) test certificate.
English language test validity
Other international entry requirements
Application and selection
How to apply
As there is high demand for this course we operate a staged admissions process with selection deadlines throughout the year.
Due to the competition for places, we give preference to students with grades above our minimum entry requirements. If we make you an offer, you will have 3 weeks in which to accept. Any offers not accepted within 3 weeks will be withdrawn so that an offer can be made to another candidate.
Please find more information in our Application and Selection section .
Staged admissions
As there is high demand for this course we operate a staged admissions process with selection deadlines throughout the year.Due to the competition for places, we give preference to students with grades above our minimum entry requirements.
If we make you an offer, you will have 3 weeks in which to accept. Any offers not accepted within 3 weeks will be withdrawn so that an offer can be made to another candidate.
Stage 1: Application received by 08 November 2024; Decision by 10 January 2025.
Stage 2: Application received by 03 January 2025; Decision by 07 March 2025.
Stage 3: Application received by 28 February 2025; Decision by 18 April 2025.
Stage 4: Application received by 23 May 2025; Decision by 20 June 2025.
You need to ensure that you submit your supporting documents with your application as it may delay us processing your application before the decision deadline.
Whilst we aim to give you a decision on your application by the deadline date, in some instances due to the competition for places and the volume of applications received, it may be necessary to roll your application forward to the next deadline date. If this is the case we will let you know after the deadline date.
Applications received after our final selection deadline will be considered at our discretion if places are still available.
Please note: All places are subject to availability and if you apply at one of the later stages, some courses may already be closed. We therefore recommend that you apply early in the cycle to secure your place with us.
Applicants who are made a conditional offer of a place must demonstrate that they have met all the conditions of their offer by 31 July 2025.
Advice to applicants
- Latest transcripts of study showing all modules completed including credit weighting and grades achieved (and English translation if applicable).
-
If you have not yet graduated, a list of the modules that you will be completing in the final year of your degree together with their credit weighting.
-
An official document from your university verifying your current weighted average mark if this information is not included in your transcript of study. Please note : this must be recorded as a percentage, not as a GPA grade, and must give the weighted average mark, not an arithmetic average mark.
-
We require references from two people who have knowledge of your academic ability in support of your application, although we can process your application with one academic reference. In most cases, these should be academic references, from a lecturer or professor at your last university. In some cases (for example, if your academic studies occurred some time ago), it might be more appropriate to submit recommendations from those familiar with your professional experience. If you have difficulty in identifying suitable referees you should ask for advice from the admissions team for your course. We will contact your referees directly after you submit your application and direct them to complete our online reference form.
-
Degree certificate if you have already graduated (and English translation if applicable).
-
Curriculum vitae (CV) if you graduated more than three years ago.
- Personal Statement.
-
If English is not your first language, we also require proof of your English language ability. If you have already taken an English language qualification, please include your certificate with your application. We may be willing to consider your application without this document, but if we choose to make you an offer, the conditions will include IELTS (or equivalent qualification).
You must submit all these supporting documents with your application. If any of the above information is missing, we will not be able to consider your application and it may be rolled forward to the next stage or withdrawn.
How your application is considered
We consider your full academic history including which course units you have taken and the marks obtained. Even if you have met our minimum entry requirements, we will take into account your marks in relevant course units in our final decision making.
If you graduated more than three years ago, we will also consider the information contained on your CV and any relevant work experience you have to assess if you are still able to fulfil the entry criteria.
Interview requirements
Overseas (non-UK) applicants
Self-funded international applicants for this course will be required to pay a deposit of £1,000 towards their tuition fees before a confirmation of acceptance for studies (CAS) is issued. This deposit will only be refunded if immigration permission is refused. We will notify you about how and when to make this payment.
Please upload a copy of your current valid passport with your application showing the photograph page with your application. For CAS purposes, this must show your full name, date of birth, nationality, passport number and the date the passport is valid until, which must be later than the date of your planned arrival in the UK, and the start date of your course.
If you have previously studied in the UK on a Tier 4 visa as an undergraduate or postgraduate student, please send a copy of your previous CAS statement to us as it will assist with the issue of your new CAS statement. This includes study in the UK on study abroad programmes and any study that you did not complete.
You cannot use your CAS to apply for a visa more than three months before the start date of your course. The Admissions Team will contact you at the appropriate time.
Your CAS number is only valid for one Tier 4 application.
Deferrals
Applications for deferred entry are not accepted for this course. If you receive an offer for 2024 entry and decide not to accept it, should you subsequently wish to be considered for 2025 entry you would be required to reapply.
Re-applications
Course details
Course description
The Probability and Statistics group in the Department of Mathematics have a long-standing reputation of offering one-year, high-quality taught courses in areas of Statistics leading to the degree of MSc. These courses have aimed to offer a thorough professional training which prepare students to embark on statistical careers in a variety of areas.
There is a shortage of statisticians trained to postgraduate level in the UK, meaning that you will be a highly desired candidate upon completion of this MSc. They have also provided a very good foundation for further study at PhD level.
Coursework and assessment
There are two teaching semesters of 12 weeks each and approximately 15 weeks of dissertation work. Assessment for the taught part is by exams and coursework. Following the successful completion of the taught part (worth a total of 120 credits) students are then expected to work on a dissertation from June to September which is worth a further 60 credits, making 180 credits in total. Information on the various topics and projects which will be available for dissertation are provided to the students in May from which they are invited to state their preferences.
Course unit details
The taught part of the programme is divided into two 12-week semesters, each followed by a two- or three-week period of examinations. This in turn is followed by a period of approximately 12 weeks of research work over the summer which is supervised by a member of academic staff and ends with submission of the MSc dissertation in September. Full-time students attend weekly lectures and support classes for four modules (4 x 15 credits) in each semester. Students can also enrol on a part-time basis. In this case they study over a two year period and only take two modules per semester, with the dissertation being completed at the end of the second year. Details of the programme structure are given below, which are subject to review and continual improvement.
Main Programme
Semester One:
- Linear Models with Nonparametric Regression
- Statistical Computing
- Statistical Inference
- Multivariate Statistics
Semester Two:
- Generalized Linear Models and Survival Analysis
- Longitudinal Data Analysis
- Markov Chain Monte Carlo (MCMC)
- Design and Analysis of Experiments
This degree programme is accredited by the Royal Statistical Society.
Accreditation by the Royal Statistical Society (RSS) provides reassurance that our MSc programme produces graduates with the technical skills and subject knowledge required of a statistician. This provides our graduates with a competitive edge in the job market and provides employers with an assurance of quality of our degree.
Dissertation: Following the successful completion of the taught part of the programme (worth a total of 120 credits) students are then expected to work on a dissertation from June to September which is worth a further 60 credits, making 180 credits in total. Information on the various topics and projects which will be available for dissertation are provided to the students in May from which they are invited to state their preferences.
Course unit list
The course unit details given below are subject to change, and are the latest example of the curriculum available on this course of study.
Title | Code | Credit rating | Mandatory/optional |
---|---|---|---|
MSc Dissertation (60cr) Statistics | MATH60000S | 60 | Mandatory |
Linear Models with Nonparametric Regression | MATH68011 | 15 | Mandatory |
Generalised Linear Models and Survival Analysis | MATH68052 | 15 | Mandatory |
Multivariate Statistics | MATH68061 | 15 | Mandatory |
Design and Analysis of Experiments | MATH68082 | 15 | Mandatory |
Statistical Theory | MATH68201 | 15 | Mandatory |
Bayesian Statistics | MATH68221 | 15 | Mandatory |
Time Series Analysis and Forecasting | MATH68032 | 15 | Optional |
Longitudinal and Spatial Data Analysis | MATH68132 | 15 | Optional |
Statistical Machine Learning | MATH68292 | 15 | Optional |
Scholarships and bursaries
Across our institution, we offer a number of postgraduate taught scholarships and awards to outstanding UK and international students each year. We are one of the Martingale Foundation's new university partners. The Martingale Foundation helps provide access to postgraduate mathematics study for UK students facing financial barriers by offering fully-funded MSc and PhD programmes. Information about eligibility is available via the Martingale Foundation website and applications will open on 13 August 2025. The deadline to apply is Sunday 19 October 2025.
The University of Manchester is committed to widening participation in master's study, and allocates £300,000 in funding each year. Our Manchester Masters Bursaries are aimed at widening access to master's courses by removing barriers to postgraduate education for students from underrepresented groups.
For more information, see the Department of Mathematics Fees and funding page or visit the University of Manchester funding for master's courses website for more information.
If you are a sponsored student please check the requirements of your sponsorship before registering on a course. If you require further information please contact the Department.
Facilities
The Department of Mathematics is the largest in the UK with an outstanding research reputation and facilities .
Disability support
Careers
Career opportunities
This course will prepare students for a broad range of statistical careers, particularly in the financial, medical, pharmaceutical and industrial sectors of the economy, but also with local and national government agencies, as well as in other areas. It will also provide an excellent foundation for students wishing to pursue advanced postgraduate research in statistics.
Upon completion of this MSc, you will be a highly desired candidate. Not only is there a shortage of statisticians trained to postgraduate level in the UK, but The University of Manchester is one of the most targeted university by graduate employers*.
See below just some of the place our MSc Statistics graduates are employed:
- Hellenic Bank
- University of Bristol
- Simmondley Dental Practice
- App Annie
- Micro-Pensions Systems
And below you can find some of the roles that they do:
- Lecturer
- Biostatistician
- Data Analyst
- Project Manager
- Mathematics Teacher
Accrediting organisations
This degree programme is accredited by the Royal Statistical Society.
Accreditation by the Royal Statistical Society (RSS) provides reassurance that our MSc programme produces graduates with the technical skills and subject knowledge required of a statistician. This provides our graduates with a competitive edge in the job market and provides employers with an assurance of quality of our degree.